AI IN ORTHODONTICS: DIAGNOSTICS AND TREATMENT PLANNING
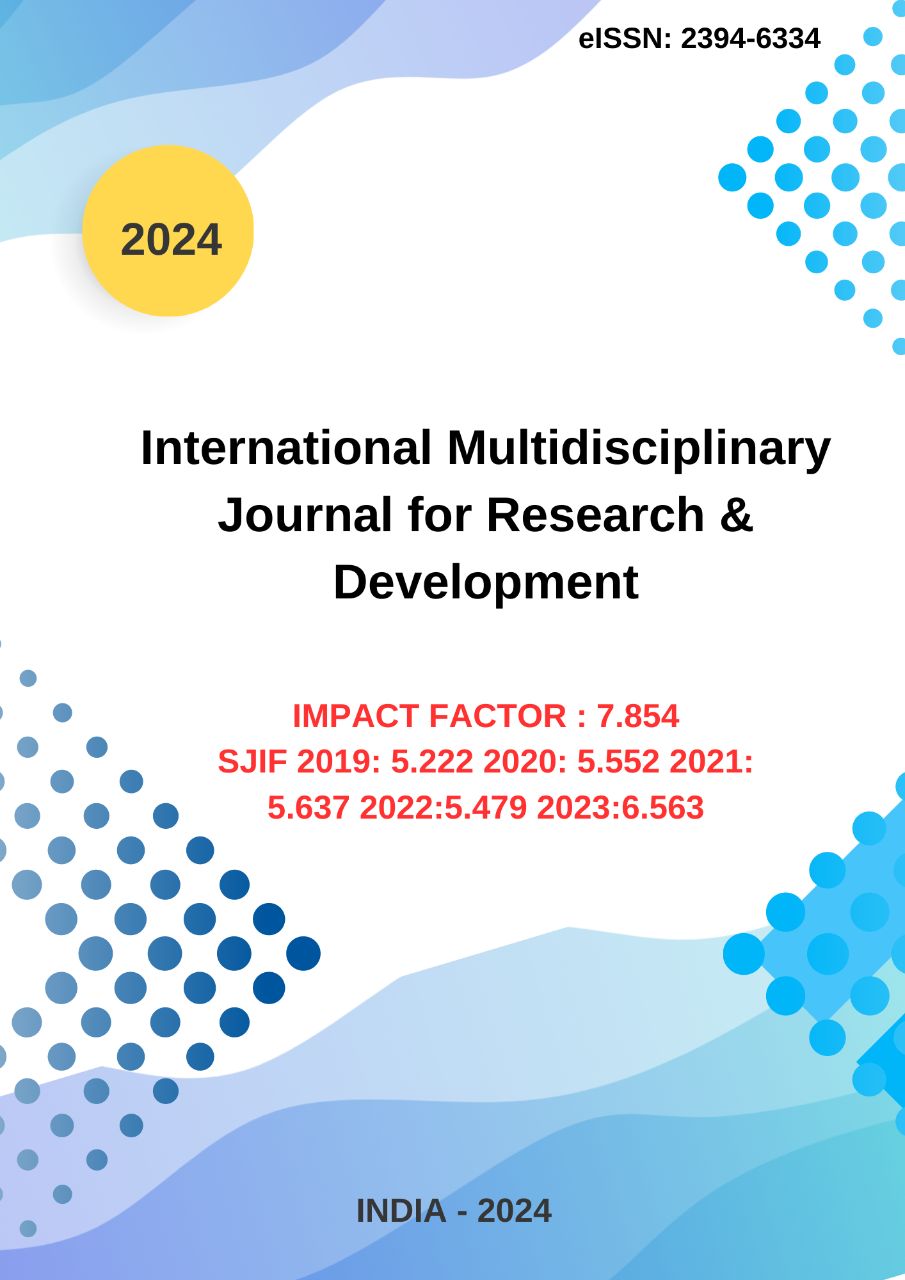
Abstract
Artificial Intelligence (AI) is revolutionizing the field of orthodontics, offering enhanced diagnostic precision and improved treatment planning. By integrating AI into orthodontic practices, professionals can leverage advanced algorithms for detailed dental diagnostics, accurate cephalometric analysis, and reliable skeletal age assessments. AI's role in evaluating the temporomandibular joint (TMJ) and aiding in clinical decision-making processes further underscores its significance in modern orthodontics. Moreover, AI facilitates patient telemonitoring, allowing for continuous care and monitoring outside traditional clinical settings. Despite these advancements, the heterogeneity of studies and the inherent complexity of AI algorithms necessitate a cautious approach. Manual oversight remains essential to ensure the reliability of AI-driven conclusions. Furthermore, the ethical and privacy considerations associated with AI deployment must be meticulously managed. As AI continues to evolve, its integration into orthodontic practice demands ongoing learning, stringent governance, and a commitment to addressing these critical concerns to realize its full potential and maintain the trust of practitioners and patients alike.
Keywords
Orthodontics; artificial intelligence; deep learning; cephalometric analysis; radiology; CBCT; skeletal age; treatment planning.
References
- McCarthy, J.; Minsky, M.L.; Rochester, N.; Shannon, C.E. A Proposal for the Dartmouth Summer Research Project on Artificial Intelligence. AI Mag. 2006, 27, 12.
- Haenlein, M.; Kaplan, A. A Brief History of Artificial Intelligence: On the Past, Present, and Future of Artificial Intelligence. Calif. Manag. Rev. 2019, 61, 5–14. [CrossRef]
- Schwendicke, F.; Golla, T.; Dreher, M.; Krois, J. Convolutional Neural Networks for Dental Image Diagnostics: A Scoping Review. J. Dent. 2019, 91, 103226. [CrossRef] [PubMed]
- Esteva, A.; Kuprel, B.; Novoa, R.A.; Ko, J.; Swetter, S.M.; Blau, H.M.; Thrun, S. Dermatologist-Level Classification of Skin Cancer with Deep Neural Networks. Nature 2017, 542, 686. [CrossRef] [PubMed]
- Gulshan, V.; Peng, L.; Coram, M.; Stumpe, M.C.; Wu, D.; Narayanaswamy, A.; Venugopalan, S.; Widner, K.; Madams, T.; Cuadros, J.; et al. Development and Validation of a Deep Learning Algorithm for Detection of Diabetic Retinopathy in Retinal Fundus Photographs. JAMA 2016, 316, 2402–2410. [CrossRef]
- Mazurowski, M.A.; Buda, M.; Saha, A.; Bashir, M.R. Deep Learning in Radiology: An Overview of the Concepts and a Survey of the State of the Art with Focus on MRI. J. Magn. Reson. Imaging 2019, 49, 939–954. [CrossRef]
- Saida, T.; Mori, K.; Hoshiai, S.; Sakai, M.; Urushibara, A.; Ishiguro, T.; Satoh, T.; Nakajima, T. Differentiation of Carcinosarcoma
- from Endometrial Carcinoma on Magnetic Resonance Imaging Using Deep Learning. Pol. J. Radiol. 2022, 87, 521–529. [CrossRef]
- McNabb, N.K.; Christensen, E.W.; Rula, E.Y.; Coombs, L.; Dreyer, K.;Wald, C.; Treml, C. Projected Growth in FDA-Approved Artificial Intelligence Products Given Venture Capital Funding. J. Am. Coll. Radiol. 2023. [CrossRef]
- Pianykh, O.S.; Langs, G.; Dewey, M.; Enzmann, D.R.; Herold, C.J.; Schoenberg, S.O.; Brink, J.A. Continuous Learning AI in Radiology: Implementation Principles and Early Applications. Radiology 2020, 297, 6–14. [CrossRef]
- Milam, M.E.; Koo, C.W. The Current Status and Future of FDA-Approved Artificial Intelligence Tools in Chest Radiology in the United States. Clin. Radiol. 2023, 78, 115–122. [CrossRef]
- Giełczyk, A.; Marciniak, A.; Tarczewska, M.; Kloska, S.M.; Harmoza, A.; Serafin, Z.; Wo´zniak, M. A Novel Lightweight Approach to COVID-19 Diagnostics Based on Chest X-Ray Images. J. Clin. Med. 2022, 11, 5501. [CrossRef] [PubMed]
- Kloska, A.; Tarczewska, M.; Giełczyk, A.; Kloska, S.M.; Michalski, A.; Serafin, Z.; Wo´zniak, M. Influence of Augmentation on the Performance of the Double ResNet-Based Model for Chest X-Ray Classification. Pol. J. Radiol. 2023, 88, 244–250. [CrossRef] [PubMed]
- Fujima, N.; Kamagata, K.; Ueda, D.; Fujita, S.; Fushimi, Y.; Yanagawa, M.; Ito, R.; Tsuboyama, T.; Kawamura, M.; Nakaura, T.; et al. Current State of Artificial Intelligence in Clinical Applications for Head and Neck MR Imaging. Magn. Reson. Med. Sci. 2023, 22, 401–414. [CrossRef] [PubMed]
- Matsubara, K.; Ibaraki, M.; Nemoto, M.; Watabe, H.; Kimura, Y. A Review on AI in PET Imaging. Ann. Nucl. Med. 2022, 36, 133–143. [CrossRef] [PubMed]
- Wang, B.; Jin, S.; Yan, Q.; Xu, H.; Luo, C.;Wei, L.; Zhao,W.; Hou, X.; Ma,W.; Xu, Z.; et al. AI-Assisted CT Imaging Analysis for
- COVID-19 Screening: Building and Deploying a Medical AI System. Appl. Soft Comput. 2021, 98, 106897. [CrossRef] [PubMed]
- Bichu, Y.M.; Hansa, I.; Bichu, A.Y.; Premjani, P.; Flores-Mir, C.; Vaid, N.R. Applications of Artificial Intelligence and Machine Learning in Orthodontics: A Scoping Review. Prog. Orthod. 2021, 22, 18. [CrossRef]
- Williams, M.; Haugeland, J. Artificial Intelligence: The Very Idea. Technol. Cult. 1987, 28, 706. [CrossRef]
- Schwartz, W.B.; Patil, R.S.; Szolovits, P. Artificial Intelligence in Medicine. Where Do We Stand? N. Engl. J. Med. 1987, 316,
- –688. [CrossRef]
- Faber, J.; Faber, C.; Faber, P. Artificial Intelligence in Orthodontics. APOS Trends Orthod. 2019, 9, 201–205. [CrossRef]
- Bishop, C. Pattern Recognition and Machine Learning; Springer: Berlin/Heidelberg, Germany, 2007; Volume 16.
- Rajpurkar, P.; Irvin, J.; Ball, R.L.; Zhu, K.; Yang, B.; Mehta, H.; Duan, T.; Ding, D.; Bagul, A.; Langlotz, C.P.; et al. Deep Learning for Chest Radiograph Diagnosis: A Retrospective Comparison of the CheXNeXt Algorithm to Practicing Radiologists. PLoS Med. 2018, 15, e1002686. [CrossRef]
- Chilamkurthy, S.; Ghosh, R.; Tanamala, S.; Biviji, M.; Campeau, N.G.; Venugopal, V.K.; Mahajan, V.; Rao, P.; Warier, P. Deep
- Learning Algorithms for Detection of Critical Findings in Head CT Scans: A Retrospective Study. Lancet 2018, 392, 2388–2396. [CrossRef] [PubMed]
- Yu, A.C.; Mohajer, B.; Eng, J. External Validation of Deep Learning Algorithms for Radiologic Diagnosis: A Systematic Review.
- Radiol. Artif. Intell. 2022, 4, e210064. [CrossRef] [PubMed]
- Wu, N.; Phang, J.; Park, J.; Shen, Y.; Huang, Z.; Zorin, M.; Jastrzebski, S.; Fevry, T.; Katsnelson, J.; Kim, E.; et al. Deep Neural
- Networks Improve Radiologists’ Performance in Breast Cancer Screening. IEEE Trans. Med. Imaging 2020, 39, 1184–1194. [CrossRef] [PubMed]
- Kuo, W.; H‥аne, C.; Mukherjee, P.; Malik, J.; Yuh, E.L. Expert-Level Detection of Acute Intracranial Hemorrhage on Head Computed Tomography Using Deep Learning. Proc. Natl. Acad. Sci. USA 2019, 116, 22737–22745. [CrossRef] [PubMed] J. Clin. Med. 2024, 13, 344 13 of 19
- Bulten, W.; Pinckaers, H.; van Boven, H.; Vink, R.; de Bel, T.; van Ginneken, B.; van der Laak, J.; Hulsbergen-van de Kaa, C.;
- Litjens, G. Automated Deep-Learning System for Gleason Grading of Prostate Cancer Using Biopsies: A Diagnostic Study. Lancet
- Oncol. 2020, 21, 233–241. [CrossRef] [PubMed]
- Hosny, A.; Aerts, H.J.; Mak, R.H. Handcrafted versus Deep Learning Radiomics for Prediction of Cancer Therapy Response. Lancet Digit. Health 2019, 1, e106–e107. [CrossRef]
- Lou, B.; Doken, S.; Zhuang, T.; Wingerter, D.; Gidwani, M.; Mistry, N.; Ladic, L.; Kamen, A.; Abazeed, M.E. An Image-Based
- Deep Learning Framework for Individualising Radiotherapy Dose: A Retrospective Analysis of Outcome Prediction. Lancet Digit. Health 2019, 1, e136–e147. [CrossRef]
- Haug, C.J.; Drazen, J.M. Artificial Intelligence and Machine Learning in Clinical Medicine, 2023. New Engl. J. Med. 2023, 388, 1201–1208. [CrossRef]
- Vandenberghe, B.; Jacobs, R.; Bosmans, H. Modern Dental Imaging: A Review of the Current Technology and Clinical Applications
- in Dental Practice. Eur. Radiol. 2010, 20, 2637–2655. [CrossRef]